Logistic Regression & Least Square Probability Classification
1. Logistic Regression
Likelihood function, as interpreted by wikipedia:
plays one of the key roles in statistic inference, especially methods of estimating a parameter from a set of statistics. In this article, we’ll make full use of it.
Pattern recognition works on the way that learning the posterior probability
p(y|x) of pattern
x belonging to class
y. In view of a pattern
x, when the posterior probability of one of the class
y achieves the maximum, we can take
x for class
y, i.e.
The posterior probability can be seen as the credibility of model
x belonging to class
y.
In Logistic regression algorithm, we make use of linear logarithmic function to analyze the posterior probability:
q(y|x,θ)=exp(∑bj=1θ(y)jϕj(x))∑cy′=1exp(∑bj=1θ(y′)jϕj(x)) Note that the denominator is a kind of regularization term. Then the Logistic regression is defined by the following optimal problem:
We can solve it by gradient descent method:
- Initialize θ.
- Pick up a training sample (xi,yi) randomly.
- Update θ=(θ(1)T,…,θ(c)T)T along the direction of gradient ascent:
θ(y)←θ(y)+ϵ∇yJi(θ),y=1,…,c where ∇yJi(θ)=−exp(θ(y)Tϕ(xi))ϕ(xi)∑cy′=1exp(θ(y′)Tϕ(xi))+{ ϕ(xi)0(y=yi)(y≠yi) - Go back to step 2,3 until we get a θ of suitable precision.
Take the Gaussian Kernal Model as an example:
q(y|x,θ)∝exp⎛⎝∑j=1nθjK(x,xj)⎞⎠ Aren’t you familiar with Gaussian Kernal Model? Refer to this article:
Here are the corresponding MATLAB codes:
n=90; c=3; y=ones(n/c,1)*(1:c); y=y(:);x=randn(n/c,c)+repmat(linspace(-3,3,c),n/c,1);x=x(:);hh=2*1^2; t0=randn(n,c);for o=1:n*1000 i=ceil(rand*n); yi=y(i); ki=exp(-(x-x(i)).^2/hh); ci=exp(ki'*t0); t=t0-0.1*(ki*ci)/(1+sum(ci)); t(:,yi)=t(:,yi)+0.1*ki; if norm(t-t0)<0.000001 break; end t0=t;endN=100; X=linspace(-5,5,N)';K=exp(-(repmat(X.^2,1,n)+repmat(x.^2',N,1)-2*X*x')/hh);figure(1); clf; hold on; axis([-5,5,-0.3,1.8]);C=exp(K*t); C=C./repmat(sum(C,2),1,c);plot(X,C(:,1),'b-');plot(X,C(:,2),'r--');plot(X,C(:,3),'g:');plot(x(y==1),-0.1*ones(n/c,1),'bo');plot(x(y==2),-0.2*ones(n/c,1),'rx');plot(x(y==3),-0.1*ones(n/c,1),'gv');legend('q(y=1|x)','q(y=2|x)','q(y=3|x)');
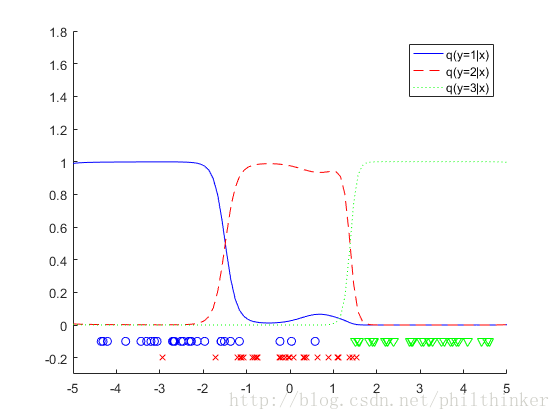
2. Least Square Probability Classification
In LS probability classifiers, linear parameterized model is used to express the posterior probability:
q(y|x,θ(y))=∑j=1bθ(y)jϕj(x)=θ(y)Tϕ(x),y=1,…,c These models depends on the parameters
θ(y)=(θ(y)1,…,θ(y)b)T correlated to each classes
y that is diverse from the one used by Logistic classifiers. Learning those models means to minimize the following quadratic error:
Jy(θ(y))==12∫(q(y|x,θ(y))−p(y|x))2p(x)dx12∫q(y|x,θ(y))2p(x)dx−∫q(y|x,θ(y))p(y|x)p(x)dx+12∫p(y|x)2p(x)dx where
p(x) represents the probability density of training set
{ xi}ni=1.
By the Bayesian formula,
p(y|x)p(x)=p(x,y)=p(x|y)p(y) Hence
Jy can be reformulated as
Jy(θ(y))=12∫q(y|x,θ(y))2p(x)dx−∫q(y|x,θ(y))p(x|y)p(y)dx+12∫p(y|x)2p(x)dx Note that the first term and second term in the equation above stand for the mathematical expectation of
p(x) and
p(x|y) respectively, which are often impossible to calculate directly. The last term is independent of
θ and thus can be omitted.
Due to the fact that
p(x|y) is the probability density of sample
x belonging to class
y, we are able to estimate term 1 and 2 by the following averages:
1n∑i=1nq(y|xi,θ(y))2,1ny∑i:yi=yq(y|xi,θ(y))p(y) Next, we introduce the regularization term to get the following calculation rule:
J^y(θ(y))=12n∑i=1nq(y|xi,θ(y))2−1ny∑i:yi=yq(y|xi,θ(y))+λ2n∥θ(y)∥2 Let
π(y)=(π(y)1,…,π(y)n)T and
π(y)i={ 1(yi=y)0(yi≠y), then
J^y(θ(y))=12nθ(y)TΦTΦθ(y)−1nθ(y)TΦTπ(y)+λ2n∥θ(y)∥2 .
Therefore, it is evident that the problem above can be formulated as a
convex optimization problem, and we can get the analytic solution by setting the twice order derivative to zero:
.
In order not to get a negative estimation of the posterior probability, we need to add a constrain on the negative outcome:
p^(y|x)=max(0,θ^(y)Tϕ(x))∑cy′=1max(0,θ^(y′)Tϕ(x)) We also take Gaussian Kernal Models for example:
n=90; c=3; y=ones(n/c,1)*(1:c); y=y(:);x=randn(n/c,c)+repmat(linspace(-3,3,c),n/c,1);x=x(:);hh=2*1^2; x2=x.^2; l=0.1; N=100; X=linspace(-5,5,N)';k=exp(-(repmat(x2,1,n)+repmat(x2',n,1)-2*x*(x'))/hh);K=exp(-(repmat(X.^2,1,n)+repmat(x2',N,1)-2*X*(x'))/hh);for yy=1:c yk=(y==yy); ky=k(:,yk); ty=(ky'*ky +l*eye(sum(yk)))\(ky'*yk); Kt(:,yy)=max(0,K(:,yk)*ty);endph=Kt./repmat(sum(Kt,2),1,c);figure(1); clf; hold on; axis([-5,5,-0.3,1.8]);C=exp(K*t); C=C./repmat(sum(C,2),1,c);plot(X,C(:,1),'b-');plot(X,C(:,2),'r--');plot(X,C(:,3),'g:');plot(x(y==1),-0.1*ones(n/c,1),'bo');plot(x(y==2),-0.2*ones(n/c,1),'rx');plot(x(y==3),-0.1*ones(n/c,1),'gv');legend('q(y=1|x)','q(y=2|x)','q(y=3|x)');
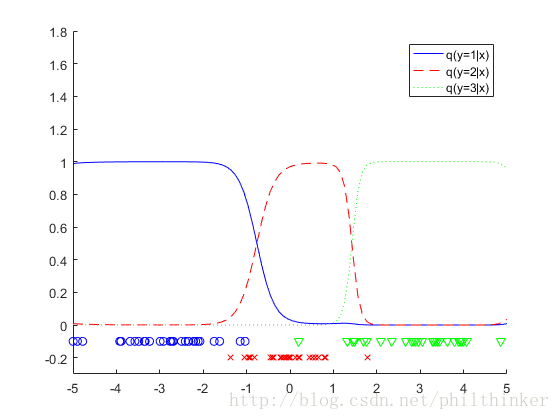
3. Summary
Logistic regression is good at dealing with sample set with small size since it works in a simple way. However, when the number of samples is large to some degree, it is better to turn to the least square probability classifiers.